Top Payer Concerns, Opportunities Around Generative AI Integration
Payers should create a unified approach to AI overall, thoroughly document generative AI integration, and ensure the explainability of their generative AI tools.
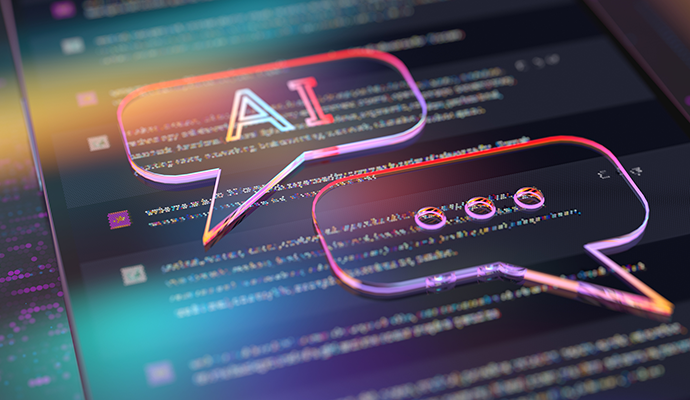
Source: Getty Images
- Generative AI may become a fixture of the health insurance landscape, seamlessly integrated into common payer processes, reducing timelines from hours or days to minutes. But for now, the tool’s future is fraught with challenges.
Care delivery transformation, clinical productivity measures, administrative simplification, and technology enablement could result in between $1 and 1.5 trillion in improvement potential by 2027, according to McKinsey & Company. Artificial intelligence—specifically, generative AI—could speed the system’s progress toward that goal.
Bringing generative AI into the health insurance system will require regulation, coordination, and innovation. If executed well, the tool could transform health insurance—and healthcare at large—not only by reducing the amount of time certain tasks may take, but potentially by decreasing costs as well.
Listen to the full podcast to hear more details. And don’t forget to subscribe on iTunes, Spotify, or Google Podcasts.
Ginny Whitman, senior manager of public policy at the Alliance of Community Health Plans (ACHP), shared on Healthcare Strategies how payers have responded to the tool and what they can do to move forward in the right direction.
Difference between traditional payer AI, generative AI
The primary distinguishing factor between traditional AI and generative AI is the latter’s responsiveness and originality. Traditional—or “conversational”—AI encompasses models designed to master a certain function. It is limited by the data inputs and the task it was algorithmically destined to fulfill. Alexa and Siri, which have been around for almost a decade or more, are examples.
Meanwhile, generative AI is more adaptable. With a large enough data set—which, necessarily, is massive—these models can learn and predict patterns and create new content. The model can create images, audio, and other outputs. Examples include ChatGPT, Google Bard, and Jasper AI.
Generative AI has by no means rendered traditional AI useless. Traditional AI is well-suited for and widely used in customer service. And some tools, including ChatGPT, integrate conversational and generative AI capabilities to facilitate personalized engagement.
“It’s important when we're talking about AI for payers. A lot of payers have been doing artificial intelligence, have that integrated within their systems for years. It’s not new. What's new is generative AI. And so that's the thing that is causing all this buzz. That's the thing that's causing all this anxiety,” Whitman explained.
“We can collectively say that they recognize AI has value, they’ve been using it. It’s just this generative AI piece that is causing the concern. And so, ACHP is doing what we can to assess where our members are, what they're thinking about, what they’re concerned about, and how we can help them from a regulatory and legislative perspective.”
General concerns
Unsurprisingly, protecting health IT security while using AI tools is a major concern among healthcare stakeholders at large and health insurers particularly, who handle a lot of sensitive patient data. Any time a model touches patient data, security is a top concern.
There are also concerns that an uncoordinated approach to AI in the healthcare sector will lead to further fragmentation in an industry that already struggles with siloes.
Another woe among health insurance leaders is the lack of clear regulation around AI tools. Although many bills have been proposed, insurers still do not have definitive guidelines for using AI tools.
“There's a lot of interest right now in regulating AI very generally. But the healthcare industry is different and it always will be different for so many reasons. And so, what does regulation for AI as a whole mean for the healthcare industry, and how can we separate that out in a way that makes sense without creating the fragmentation of regulations that is so inherent to what we see in the healthcare system today?” Whitman explained.
One facet that may be a concern in other arenas but is less troubling for payers at the moment is AI hallucinations. When an AI model responds to a query falsely, it is said to be “hallucinating.” The results may include grammatical, factual, or prompt-related contradictions or may be simply irrelevant. Whitman indicated that careful design of generative AI tools can avoid or reduce these errors.
Opportunities for payers
While the challenges are plentiful, there are several potential benefits to implementing generative AI in the health insurance industry, making the tool impossible to set aside.
Generative AI may be most useful in situations that require sifting through large amounts of data. In such circumstances, the tool can create summaries of important information. Potential use cases include administrative, corporate, and to some extent clinical interactions.
Generative AI-powered member service platforms, including claim denial resolutions and benefits information queries, can improve members’ experiences and answer their questions more quickly. It could also be useful for increasing the efficiency of a contentious health insurance process: prior authorizations.
“Payers are starting to leverage generative AI to reduce costs and improve risk management and member engagement,” a Boston Consulting Group (BCG) article stated.
One validated generative AI product that BCG noted automates facets of underwriting and the claims management process. The tool analyzes cases in minutes and isolates many more significant data points compared to human reviewers, according to the platform’s website.
Another company that BCG highlighted might provide generative AI-powered predictive analytics opportunities for payers. The company’s tool helps predict high-risk patients using demographic, medical history, and social determinants of health data.
In the future, generative AI could help payers craft personalized messaging to improve member engagement.
The future of AI regulation
The problem with AI regulation at the moment, according to Whitman, is the overabundance of proposed frameworks and legislation, leading to a fragmented approach.
At the federal level, the White House issued a Blueprint for an AI Bill of Rights, which touched on the right to safe and effective systems, protections against algorithmic discrimination, and data privacy among other points. Whitman also mentioned recommendations developed by individual departments such as the Food and Drug Administration’s (FDA) guidance published in April 2023.
In the legislative branch, both Republicans and Democrats have put forward legislation to control and oversee AI-related content.
For example, in October 2023, US Senators John Kennedy (R-LA) and Brian Schatz (D-HI) introduced legislation on creating more transparency around AI-generated content, following a Senate bill to protect against discrimination and a Senate bipartisan framework introduced the previous month.
On the state level, around 12 states have enacted legislation related to artificial intelligence and 13 states have proposed legislation, according to a tracker from the law firm Bryan Cave Leighton Paisner LLP. One state has both proposed and currently enacted legislation (New York).
In the five months between May 1 and October 10, 2023, the House and Senate put forward 39 proposed bills related to artificial intelligence, according to the Brennan Center for Justice's Artificial Intelligence Legislation Tracker. Based on the Federal Register as of October 30, 2023, not a single proposed bill on the subject had been passed. In fact, none progressed beyond introduction.
Without more legislative coordination, AI innovation will slow down, Whitman warned.
One of the barriers to legislative coordination is terminology. One policymaker might base her AI proposal on regulating generative AI specifically, while another might forge a policy that refers to “artificial intelligence” as inclusive of machine learning and deep learning. Meanwhile, yet another policymaker might base his framework on regulating algorithms.
If policymakers start regulating at the algorithmic level, innovation is likely to stall.
“When you're regulating just plain algorithms because you're so scared about generative AI, you're taking us back to the stone ages and you're taking away all these tools and all these innovations and all these essential things that we need in a healthcare system,” Whitman said.
Despite the impetus and unified desire to quickly formulate guidelines for this technology, regulatory hurdles could encumber the process, both from lack of progress and from overregulating.
Whitman did not expect to see AI-related regulations pass any time soon.
The tale of two payer reactions
Given these challenges and the regulatory uncertainties, it is no surprise that payers find themselves divided on how to proceed with generative AI tools.
After engaging in conversations with payers around generative AI and seeing the conversations taking place in this industry, Whitman said that most payers fall into one of two camps.
On the one hand, there are the early adopters. Witnessing the opportunities generative AI presents, some health plans are eager to take action and test out how these tools can improve processes. These payers are piloting large language models for AI.
For example, Highmark Health (Highmark) has been piloting a Google Cloud generative AI tool that offers a number of solutions for providers and payers, called Vertex AI Search. The tool has been employed across the company, Highmark Health officials told a local news outlet. Highmark has incorporated Vertex AI Search into its app to help members track their bills, assess their health savings account balances, and check their deductibles.
On the other hand, there are risk-averse payers who have been slow to adopt these tools. Health plan decisionmakers at these companies will watch as others test the boundaries of what generative AI can or cannot do before implementing it in their own companies.
“They are very concerned about AI snake oil, if you will,” said Whitman. “And so, they’re definitely taking a little bit of a slower approach, being a lot more thoughtful about what they want to integrate within their system, building in-house versus who they might want to partner with in terms of vendors and sponsors, and things like that.”
Strategies for implementing generative AI
When one payer suggested that ACHP create a risk framework around generative AI in health insurance, Whitman responded:
“What's risky today might not be risky tomorrow.”
The AI landscape in healthcare is constantly evolving and the regulations are still nascent. As a result, predicting risk on an individual payer level is difficult.
However, payers can take steps to implement generative AI in a strategic way, Whitman assured.
First, payers should construct a unified, company-wide strategy around AI overall. The National Institute of Standards and Technology (NIST) released a framework for AI risk management that could be useful.
Whitman emphasized that AI tools can be designed to serve only administrative simplification purposes. As a result, they would not touch sensitive member data, minimizing the payer’s risk. Regardless, it is important to develop pilots for new AI technologies before implementing them broadly.
Second, payers should document everything about the generative AI tools that they are developing and using. This will protect them in the case of a lawsuit. Moreover, it will improve companies’ ability to define their generative AI tools, explain how they are used, and express the intended outcomes.
“The most important thing right now is your explain-ability around the systems that you're working on,” Whitman underscored. “Can you show that it’s not a black box?”
She listed a couple of simple questions that payers can ask themselves to explain their tools.
- What are the inputs?
- What are the outputs?
- How is it functioning?
Finally, it is critical for health insurance leaders to remember artificial intelligence cannot fix everything. Efficient care coordination and strong communication between providers, payers, and members remain crucial to securing positive outcomes in generative AI-powered processes.
"The important thing to remember is AI is just a tool. Yes, it is artificial human-like intelligence, but it's human-like. It's just a tool. People are still needed in this process. There's no silver bullet that's going to have a system running insurers' plans for them,” Whitman concluded.